Coronavirus in Context: Covid-19 Prevalence
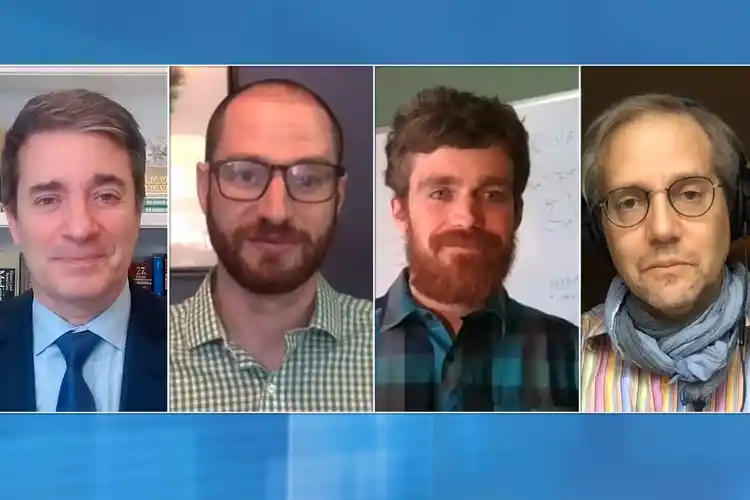
Hide Video Transcript
Video Transcript
[MUSIC PLAYING]
What if I told you that, instead of 700,000 cases of coronavirus, there is over 10 times that amount? Are we getting it all wrong? To discuss this, I have three guests. The first is Dr. Justin Silverman. He is an assistant professor at Penn State University. Dr. Nathaniel Hupert. He is an associate professor at Cornell Weill Medicine. And Dr. Alex Washburne. He is a research scientist at Montana State.
Gentlemen, thanks for joining me.
And so that's basically the data. And what we did was just some back of the envelope calculations to say, well, if we see this relative amount of ILI within the network, what does that mean in terms of the total number of people who are presenting to their physicians with influenza-like illness around the country? And what we found was that this is far in excess of what we had seen in previous years.
So really, to-- to get to the next step and say, well what it-- to link this to coronavirus we had to separate out the signal, because influenza-like illness can be due to a number of things. It can be due to influenza. It can also be due to all kinds of endemic respiratory viruses. So we separated the influenza-like illness signal into three components.
The ILI that can be attributed to influenza, using laboratory surveillance data, also maintained by the CDC. The seasonal endemic respiratory viruses. And then what was left was what we're calling the excess ILI.
So that-- however, what we found upon looking at it closer is that, actually, it seems like some providers may actually represent entire physician organizations. You know, probably not that at the scale of the whole hospital. But maybe, you know, maybe an office with multiple physicians working in them. And so because we basically we decided that we were not going to use that estimate anymore, and instead used an estimate for the total number of patients a physician sees per day based on a survey conducted by the physicians foundation a couple of years ago.
And so we thought we could add some clarity to this discussion by pointing out that, when you carefully count the number of people arriving in physicians with non flu influenza-like illness, it adds up to a really big number by early March. We point out that the only way to get to that number from the start date of January 15, which is the first known start for the onset of community transmission in the US, the only way to get from this big number in early March from a first patient in January 15, is if you're growing sufficiently fast.
And so we thought this could provide some guidance for people doing forecasting elsewhere, in terms of pointing out that it's unlikely that we have doubling times-- epidemic growth rates with doubling times slower than 3.5 days in the US.
So we originally thought to avoid making statements in our manuscript because we are using this alternative data source that needs to be tested with the more gold standard tools of zero surveys and swaps. However, if there were more cases than we currently accounted, then that would suggest a lower infection fatality rate is possible.
But broadly speaking, antibody testing fits in here because, 1, it can help us understand if our estimates of ILI are correct. But more importantly, it can be used as a gold standard to assess the true prevalence and a better estimate of the infection fatality rate. So, in addition to passports immunity that some people are talking about, so if we have some antibodies, perhaps that suggest you could, you know, be less afraid-- we-- we don't-- you know, we're not experts in that realm. But we are saying that the antibody testing can link up with the influenza-like illness.
And we're finding that, for instance, in California, our estimate for the prevalence in March 15 was about 2.1%. And recently there was a antibody test done in Palo Alto, with some concerns about exactly how representative their sample pool was. But nonetheless, they found 2.8% of people were sero prevalent in early April. And so, if you backtrack early April to mid-march, the 2.1% percent we get from ILI and the 2.8% they get from the antibody testing are fairly close and much larger than people would have thought otherwise.
Where it impacts what we're doing now is around this big fight that we see about the accuracy of models. Many of the models-- in fact, I would say most that were out there-- really took to heart this large proportion of people who were infected becoming hospitalized patients. This is where we had these very large projections about volume not only of regular hospital beds, but also intensivists care, et cetera.
A huge portion of that has come to fruition. I mean, New York City is still laboring under a very very large, you know, historically large number of people in ICUs. But the projections that this would become double or triple or more of that load haven't happened, and I think we would believe won't happen. And that's because this turns out to be a much smaller fraction of this much larger number of people infected who wind up needing to go to the hospital.
So a change in the sense of what the true prevalence is has big implications for how all of these models, which are influencing policy perhaps to a degree that has never-- huge implications. I mean, there's never been a moment in time when a smaller number of mathematicians and physicists have had a bigger influence on the course of world events than-- than in the past month.
In addition though, this has huge implications for this question of a second wave or subsequent waves. And I really want to stress that if we can't get the number for the prevalence right now, then we can't make logical, scientifically-based estimates about what's going to happen. We've all seen the graphics. We've all seen the-- the-- the-- the curves from the-- the various study groups. But those are all speculative until we know what proportion of the population at that time in the future is still susceptible to the infection.
And so what we've added to this discussion is a piece of evidence that suggests that the growth rate could be faster and potentially clinical rate lower, that we may have a lower case severity rate. And hopefully that can guide future models, especially if the sero surveys and swap surveys corroborate our estimates of prevalence.
NATHANIEL HUPERT: So one of the complications here is that all of this is occurring as facts on the ground and actual physical relationships on the ground have been changing. So we had a period of time, for example, in New York City, up until March 16, when things were basically as normal. That includes a bit of this span of time that we're talking about. Then the schools shut down. And then, about a week later, the city and the state locked down.
And so one of the things that we have to remember is that, as we see some aspects of an exponential rise, the virus-- and this is the lingo of non pharmaceutical interventions-- the point of these interventions is to put the virus in the smallest closed ended box possible. And so it can run out of steam. And so we're seeing, I think, in the numbers now, a competition between the virus running out of steam in a box and then escaping that box sometimes. And so we we we will have a very complex shape of a curve of infection during this period of time when we're somewhat locked down.
You can look at mobility data, for example, and say, well, the lockdown isn't really quite as effective as as some people give it credit for. The policy implication is really about how we unlock, how do we get out of the situation that we've put ourselves in for better or for worse. One of the other big pieces of this picture that we haven't talked about are all of the public health implications of being in a lockdown-- the loss of primary care services, lack of access to emergency departments, et cetera, for normal, for routine health issues, you know.
I work as a hospitalist at lower Manhattan hospital, and we're full on a regular basis without COVID being in the picture. So a lot of this has to do with how do we recover hospital services, not just elective surgeries, but all of the necessary hospital services that are now being pushed over to the side to deal with this emergency.
But partly what comes along with this territory of, you know, of being in a bit of the odd one out saying that there's so many people is that the idea hasn't necessarily been vetted by as many people as it needs to. So more comments, more criticism, more people helping us figure out what's going on, absolutely welcome.
JOHN WHYTE
You're watching Coronavirus in Context. I'm Dr. John White, Chief Medical Officer at WebMD. What if I told you that, instead of 700,000 cases of coronavirus, there is over 10 times that amount? Are we getting it all wrong? To discuss this, I have three guests. The first is Dr. Justin Silverman. He is an assistant professor at Penn State University. Dr. Nathaniel Hupert. He is an associate professor at Cornell Weill Medicine. And Dr. Alex Washburne. He is a research scientist at Montana State.
Gentlemen, thanks for joining me.
JUSTIN SILVERMAN
Thanks for having us on. JOHN WHYTE
Let's start with Justin. In your study, you said that there's been over 8 million cases between this period of March 8 and March 28 of this year. Normally we might say there is 100,000 cases. So how did you determine this number? JUSTIN SILVERMAN
Well, so I'd love to say that there was something really complicated. But really, we used surveillance data that was maintained by the CDC that looks at the prevalence of influenza-like illness within the US. So the way this surveillance network has been set up is that there are a number of enrolled sentinel providers throughout the US. And each week, they report to the CDC regarding the total number of patients they saw and the total number of them that had that met criteria for influenza-like illness. That's fever above 100 degrees, and either cough or runny nose. And so that's basically the data. And what we did was just some back of the envelope calculations to say, well, if we see this relative amount of ILI within the network, what does that mean in terms of the total number of people who are presenting to their physicians with influenza-like illness around the country? And what we found was that this is far in excess of what we had seen in previous years.
So really, to-- to get to the next step and say, well what it-- to link this to coronavirus we had to separate out the signal, because influenza-like illness can be due to a number of things. It can be due to influenza. It can also be due to all kinds of endemic respiratory viruses. So we separated the influenza-like illness signal into three components.
The ILI that can be attributed to influenza, using laboratory surveillance data, also maintained by the CDC. The seasonal endemic respiratory viruses. And then what was left was what we're calling the excess ILI.
JOHN WHYTE
So even though we don't have testing, or widespread testing, I should say, you attributed certain non influenza influenza-like illness to coronavirus. Is that right? JUSTIN SILVERMAN
Exactly. Right. Coronavirus also presents with influenza-like illness often. And what we found was that, starting March 8, this excess influenza-like illness signal correlated very strongly with the case counts in each state. For example, we saw that states that had the highest number of cases also had the highest excess of this excess ILI. JOHN WHYTE
Now, you've revised your estimates somewhat from your original pre publication from 28 million to about 8.7 million. Still very high, more than, you know, 80 times, 800 time, whatever. Tell us about that revision. JUSTIN SILVERMAN
So originally we were trying to get from the relative amount of this excess ILI per provider to the prevalence of coronavirus in the state. One of the-- one of the pieces of data we were using was, in the ILI data, they report the number of providers that are reporting from each state. So that-- however, what we found upon looking at it closer is that, actually, it seems like some providers may actually represent entire physician organizations. You know, probably not that at the scale of the whole hospital. But maybe, you know, maybe an office with multiple physicians working in them. And so because we basically we decided that we were not going to use that estimate anymore, and instead used an estimate for the total number of patients a physician sees per day based on a survey conducted by the physicians foundation a couple of years ago.
JOHN WHYTE
Now, I want to ask Alex a question about doubling rates, and help explain that to our audience. And your data suggests that it's greater [AUDIO OUT] 0.5 days, which are current estimates. So how do you determine that? And why does that even matter? ALEX WASHBURNE
That's a great question, John. The growth rate of the epidemic is important for forecasting how long will this last. That's crucial for understanding when will we hit a peak, or how quickly do we need to implement interventions. And there's been a lot of discussion of growth rates in the community, some people believing that it could be more than six days, doubling every six days. Some people in some places are pointing out that doubling time is much faster. And so we thought we could add some clarity to this discussion by pointing out that, when you carefully count the number of people arriving in physicians with non flu influenza-like illness, it adds up to a really big number by early March. We point out that the only way to get to that number from the start date of January 15, which is the first known start for the onset of community transmission in the US, the only way to get from this big number in early March from a first patient in January 15, is if you're growing sufficiently fast.
And so we thought this could provide some guidance for people doing forecasting elsewhere, in terms of pointing out that it's unlikely that we have doubling times-- epidemic growth rates with doubling times slower than 3.5 days in the US.
JOHN WHYTE
Now, if instead of 100,000 cases during that time, there's 8 million cases, what does that say about fatality rate? I know you didn't specifically look at that, but what's the implication. ALEX WASHBURNE
Of the cuff, if there is a bigger denominator, there's a smaller rate. However, we caution that what we're doing with this influenza-like illness has not currently been properly calibrated with epidemics, and the infection fatality rate is a very important number. If we're off by 0.1%, then that's 200,000 deaths. So we originally thought to avoid making statements in our manuscript because we are using this alternative data source that needs to be tested with the more gold standard tools of zero surveys and swaps. However, if there were more cases than we currently accounted, then that would suggest a lower infection fatality rate is possible.
JOHN WHYTE
Well, there is a big difference between 8 million and 100,000. What's the role of antibody testing here? We've been hearing a lot about that. Is anybody testing still important if, all of a sudden, we have-- you were only looking over a 3-week period. Over the span of several months, what do you think that number is in terms of the number of people that are affected? And then do you even need antibody testing at that point? ALEX WASHBURNE
So the antibody testing helps us get a more gold standard measure of who's been infected. It's important to note that it takes some time from the onset of infection to the development of antibodies that can be detected. So an antibody test today actually gives us the sense of a person was infected some time ago. Exactly how long depends on the test and its sensitivity, and other things like that. But broadly speaking, antibody testing fits in here because, 1, it can help us understand if our estimates of ILI are correct. But more importantly, it can be used as a gold standard to assess the true prevalence and a better estimate of the infection fatality rate. So, in addition to passports immunity that some people are talking about, so if we have some antibodies, perhaps that suggest you could, you know, be less afraid-- we-- we don't-- you know, we're not experts in that realm. But we are saying that the antibody testing can link up with the influenza-like illness.
And we're finding that, for instance, in California, our estimate for the prevalence in March 15 was about 2.1%. And recently there was a antibody test done in Palo Alto, with some concerns about exactly how representative their sample pool was. But nonetheless, they found 2.8% of people were sero prevalent in early April. And so, if you backtrack early April to mid-march, the 2.1% percent we get from ILI and the 2.8% they get from the antibody testing are fairly close and much larger than people would have thought otherwise.
JOHN WHYTE
Now, I want to ask Nathaniel, if your prevalence rates are even slightly, you know, accurate, there are profound policy implications. Can-- can you describe how these numbers could impact the current mitigation strategies? NATHANIEL HUPERT
Right. So there are really two ways in which the new number that-- that is being laid out in this paper, and in other papers, I should add. There was one even from mid-march from Europe that speculated about a fast growth scenario, that I think Justin and Alex have have really nicely laid-- laid down. There are implications for what's happening right now, and there are implications for what will happen as these lockdowns get lifted. And especially in both situations, implications for how political leaders and policymakers can prepare the public for what to expect. Where it impacts what we're doing now is around this big fight that we see about the accuracy of models. Many of the models-- in fact, I would say most that were out there-- really took to heart this large proportion of people who were infected becoming hospitalized patients. This is where we had these very large projections about volume not only of regular hospital beds, but also intensivists care, et cetera.
A huge portion of that has come to fruition. I mean, New York City is still laboring under a very very large, you know, historically large number of people in ICUs. But the projections that this would become double or triple or more of that load haven't happened, and I think we would believe won't happen. And that's because this turns out to be a much smaller fraction of this much larger number of people infected who wind up needing to go to the hospital.
So a change in the sense of what the true prevalence is has big implications for how all of these models, which are influencing policy perhaps to a degree that has never-- huge implications. I mean, there's never been a moment in time when a smaller number of mathematicians and physicists have had a bigger influence on the course of world events than-- than in the past month.
In addition though, this has huge implications for this question of a second wave or subsequent waves. And I really want to stress that if we can't get the number for the prevalence right now, then we can't make logical, scientifically-based estimates about what's going to happen. We've all seen the graphics. We've all seen the-- the-- the-- the curves from the-- the various study groups. But those are all speculative until we know what proportion of the population at that time in the future is still susceptible to the infection.
JOHN WHYTE
So why are all the models wrong in terms of potential prevalence? And your back of that envelope [INAUDIBLE] correct? ALEX WASHBURNE
I'll say that the modelers have done an incredible job in all fields in light of the really major uncertainty about this new virus. There are key things we don't know, and that everyone has been trying to estimate as we go. And the different models differ largely in how they interpret existing data, and which things they think are most important for guiding their model. JOHN WHYTE
But you're using a different data set. You used data differently. ALEX WASHBURNE
Correct, yeah. And many of the modelers were using initially, epidemic curves coming out of China and some early inferences coming from Italy and South Korea, those were the first glimpses we had of what happens with an epidemic out-- you know, before it really came and hit us at home. And so people were doing their best in terms of estimating the key severity rate, the infection fatality rate, and things like that. And so what we've added to this discussion is a piece of evidence that suggests that the growth rate could be faster and potentially clinical rate lower, that we may have a lower case severity rate. And hopefully that can guide future models, especially if the sero surveys and swap surveys corroborate our estimates of prevalence.
JOHN WHYTE
How many millions of people from January to now do you think have been infected with coronavirus? Because a lot of people had suggested, and you reference it in your article, we haven't had adequate testing. We haven't always had accurate testing. Many people that had presented earlier this year before we were thinking about coronavirus may have had it. This has profound implications on return to work, immunity, whether there could be herd immunity. What's your best estimate? You're saying 8 million people in a 3-week period. And this has been going on for many many weeks. JUSTIN SILVERMAN
It's probably been at least 10% of the population that's been either exposed or infected. JOHN WHYTE
And Nathaniel, how does this influence policymakers in returning to work? NATHANIEL HUPERT: So one of the complications here is that all of this is occurring as facts on the ground and actual physical relationships on the ground have been changing. So we had a period of time, for example, in New York City, up until March 16, when things were basically as normal. That includes a bit of this span of time that we're talking about. Then the schools shut down. And then, about a week later, the city and the state locked down.
And so one of the things that we have to remember is that, as we see some aspects of an exponential rise, the virus-- and this is the lingo of non pharmaceutical interventions-- the point of these interventions is to put the virus in the smallest closed ended box possible. And so it can run out of steam. And so we're seeing, I think, in the numbers now, a competition between the virus running out of steam in a box and then escaping that box sometimes. And so we we we will have a very complex shape of a curve of infection during this period of time when we're somewhat locked down.
You can look at mobility data, for example, and say, well, the lockdown isn't really quite as effective as as some people give it credit for. The policy implication is really about how we unlock, how do we get out of the situation that we've put ourselves in for better or for worse. One of the other big pieces of this picture that we haven't talked about are all of the public health implications of being in a lockdown-- the loss of primary care services, lack of access to emergency departments, et cetera, for normal, for routine health issues, you know.
I work as a hospitalist at lower Manhattan hospital, and we're full on a regular basis without COVID being in the picture. So a lot of this has to do with how do we recover hospital services, not just elective surgeries, but all of the necessary hospital services that are now being pushed over to the side to deal with this emergency.
JOHN WHYTE
And-- and much of your data can help guide this. And I want to thank all three of you for participating. Justin, you said early on when we were starting that you put this information out and these analysis out because you want comment, and you want to have discussion and debate around it. JUSTIN SILVERMAN
Yeah, I mean, when I first calculated what had been the 28 million number, and even at the 8.7 million number, I called up Alex and was just flabbergasted. I mean, it was-- it's just such a difference from I think the way I'd been thinking about it, and honestly, I think the way a lot of people had been thinking about it. But partly what comes along with this territory of, you know, of being in a bit of the odd one out saying that there's so many people is that the idea hasn't necessarily been vetted by as many people as it needs to. So more comments, more criticism, more people helping us figure out what's going on, absolutely welcome.
JOHN WHYTE
Well, thank you for coming on, all three of you, to talk about your data. NATHANIEL HUPERT
Thank you, John. JUSTIN SILVERMAN
Thank you very much. JOHN WHYTE
And thank you for watching Coronavirus in Context. I'm Dr. John White.